By Jacques Bughin
Introduction
Our history is full of big, bold investments united by their ability to mobilize resources and inspire progress and dominance, – from the building of the pyramids in Egypt and cathedrals across Europe in the Middle Age, to the US NASA programme to land on the moon in the 1960s.
Humanity’s tradition of bold investments is now seen in the pursuit of cutting-edge artificial intelligence and quantum computing, which become the next frontier for economic and technological leadership. Investment in Artificial Intelligence (AI) has grown rapidly since the 2010s, with a boom due to generativeAI and Large Language Models (LLM).
If investments in Quantum Computing (QC) started a little later than those in AI, investments are however already comparable to historic projects such as the Apollo programme or the Human Genome Project, and promise a tech that can far surpass traditional binary computing as our generation knows it.
Governments have long taken notice– the spent led by the classical suspects of China, and US might have already invested $55 billion in QC technology in the recent years , with the European Union spending about 7 billon for its Quantum Flagship initiative, and China has invested an estimated $15–$20 billion in quantum research through initiatives such as the National Laboratory for Quantum Information Sciences in Hefei.
Multiple companies have piloted the technologies with good success, including the likes of Volkswagen, Bosch, Exxon or JP Morgan. A defining moment is the intersection of Quantum with AI opening new possibilities for industries while amplifying existing quantum computing applications. Another « ah ah moment for the take off of Quantum technology is without doubt, Google showcasing its Willow chip’s ability to perform computations significantly beyond the capabilities of classical computer, with spectacular reduction in error rates. (Table 1)
Other FAANGS are not resting. Amazon Web Services, for example, offers Braket as a fully managed service platform that allows researchers and developers to design, test, and run quantum algorithms in a cloud environment; it also recently launched Quantum Embark program, a consulting service aimed at preparing customers to integrate quantum computing into their operations. Over the years, NVIDIA has also expanded its technology solutions to include the NVIDIA DGX Quantum for the development of hybrid quantum-classical computing system.
Table 1: Quantum experiments
Amidst this market evolution, CEOs face a critical question: What should their company’s quantum strategy be? Should one dive-in now, or wait for the technology to mature? How can quantum computing align with existing initiatives in artificial intelligence (AI) and other advanced technologies? The first imperative, before acting, is to come to grips with the carousel of this technology.
The Known Knowns: Quantum is Closer than you Think
1.The Quantum Leap
Quantum mechanics is related to theoretical physics, but it crossed over into engineering and computing with a couple of milestones.
The first was a lecture by Richard Feynman in may 1981 entitled “Simulating physics with computers.”, which proposed the idea of using quantum computers to simulate many-body systems that are otherwise too difficult to manipulate with classical computers. The second milestone came with the 1985 study by David Deutsch, which formalised the concept of a quantum computer and described its potential advantage over classical computers in solving problems unrelated to physics. Finally, a third milestone came with the work of Peter Shor, who developed a quantum way of performing a Fourier transform and factoring large numbers that was an order of magnitude more efficient than conventional computing.computer science with a couple of milestones.
These milestones helped define quantum computing as a technique beyond pure physics that exploits phenomena such as superposition, entanglement and quantum tunneling to process information in a fundamentally different way from classical computing. Most importantly, quantum computers use qubits, which can exist as superpositions of the two binary bits (0 or 1) used in current computing paradigms. This unique property allows quantum systems to process large amounts of information simultaneously, with computational power growing exponentially with the number of qubits.
2. Google and the Quantum Achilles heel
But while intellectually powerful, a natural question for quantum AI is whether such large-scale quantum computers could be successfully built.
Remember that, unlike classical computers, entangled qubits are interdependent, so the state of one qubit immediately affects others, and measuring a qubit also involves probabilistically collapsing its superposition into a single state, permanently altering the systems. These properties mean that quantum computation is challenging because superpositions tend to be quite fragile and decay easily (“decoherence”), while quantum computers can also be inaccurate because the quantum gates used in quantum computation require the tolerance of the computation to these inaccuracies to be large enough to allow quantum gates to be built.
While Peter Shor also went to discover quantum error-correcting codes and fault-tolerant methods for reliable quantum computation, this “accuracy threshold theorem” for quantum computing remained a practical challenge for years, until recent efforts by companies such as IBM and Google. Following major advances at its AI lab and promising early deployment of its Sycamore chip, Google has recently achieved the “« below threshold » milestone where the error rate begins to shrink exponentially with scale. This means that performance goes hand in hand with a much higher quality of quantum computing – opening the venue for a quantum computing market play.
3. AI can be the killer app
Not only was the error rate low – an improvement many times over Willow’s predecessor, Sycamore, a few years ago – but Google’s 105 physical qubit Willow processor is also a sign of unique power. In fact, the experiment delivered solutions in five minutes to problems that would otherwise take the world’s fastest supercomputer 10 septillion years to solve (Table 2)
Table 2 : Google Quantum Lab zoom
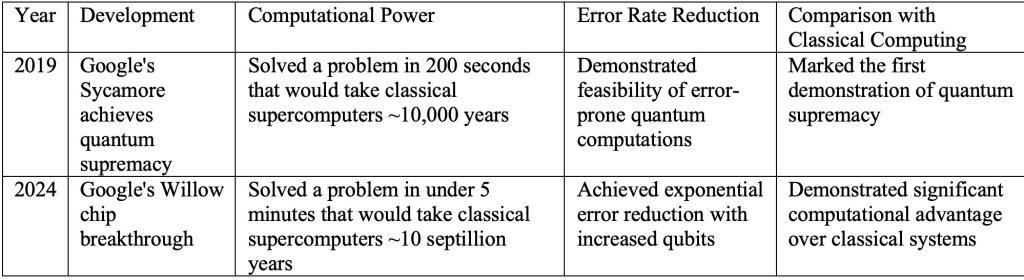
This exponential gain in computing power could be a potential game changer in an age of energy-guzzling computing needs and LLM data limitations for effective AI.
At present, one can either praise and pay the price of Nvidia GPUs for massively parallel architecture, or onez can bet on the potential of quantum for another big leap in computing. In this context, one can anticipate the potential of quantum artificial intelligence (QAI), where Quantum Machine Learning, among other things, could significantly reduce the time required for tasks such as neural network training or combinatorial problem optimization, offering exponential speedups for major complex applications such as finance, portfolio optimization and risk analysis; traffic flow management and vehicle routing in logistics, automated cars, drug discovery and simulation of molecular interactions in biotech and pharma, or still weather prediction and material design.
Furthermore, AI can support quantum progress. For example, ML can help further reduce quantum errors, as AI’s pattern recognition can be used to detect anomalies in qubit behaviour, predict noise patterns in quantum systems, and optimize quantum error correction codes.
The Known Unknowns: Show Me the Economics
1. Market potential
The current market value of QAI applications is still non-existent, with the money currently spent on QAI going to those building the infrastructure and software platforms, such as Amazon or Nvidia, and to quantum native players such as Rigetti and D-Wave, who are at the forefront of building quantum processors.
Some analysts have ventured to estimate the market potential, with an applications market (representing 15-20% of total spending) worth between USD 5-20 billion in 5 years, but accelerating dramatically thereafter. While the current cost of quantum systems and their operational complexity make them economically viable in the short term mainly for niche applications, the above estimates are likely to be inadequate for a number of reasons.
First, these estimates did not anticipate Google’s recent breakthrough with Willow, which is likely to bring the market to fruition much faster than their base case. Second, the difference between classical and quantum computing is that the latter will work much faster, reducing the time required by AI engineers. As such, the cost/performance of quantum will be driven primarily by technology, while classical AI will still have to deal with expensive engineering work, so the economics will tilt in favour of quantum AI for complex tasks rather quickly. Third, it has often been assumed that quantum AI will follow AI in adoption – but recent evidence suggests that the new technology will have accelerated adoption due to the capabilities already invested in AI and genAI. Finally, quantum AI and classical AI can work together to great market benefit in the short term, as offered as a hybrid solution. Nvidia is offering such a platform, both to protect its GPU market, but also because quantum/classical are likely to be used differently by industries.
Finally, quantum AI and classical AI can work together to great market benefit in the short term if offered as a hybrid solution. Nvidia is offering such a platform, partly to protect its GPU market, but also because quantum/classical are likely to be used differently by industries.
2. Market dynamics
Besides the technological aspects of quantum, the quantum computing market is in its formative stages, and its dynamics market will depend on a mix of demand and supply factors, including regulation.
On the demand side, the most obvious cases for quantum AI are in complex areas such as supply chain management, finance, energy and healthcare (drug discovery). While these sectors have the most to gain, industrial sectors such as energy have historically been late adopters of digital technologies.
On the supply side, the future dynamics among key players—startups, incumbents, and ecosystem facilitators—will shape how the technology is adopted and how its provision evolves.
Major Incumbents such as IBM, Google, Amazon, Nvidia and Microsoft will likely roll out their quantum platforms (such as IBM’s Q Network, Amazon’s Braket, or Microsoft Azure Quantum) that will democratize quantum computing by offering possible Saas services to quantum resources via cloud services. They are likely to interplay among themselves and regulator to define standards. Finally, those players (are likely to be offering only hybrid quantum-classical solutions (as in the case of NVIDIA’s DGX Quantum) that will blend GPU-based AI with quantum processing, as a way to control the market evolution, and make arbitrage on their legacy.
Finally, the complete market dynamics are likely to be shaped through a series of innovative technology pushes set by quantum-native start ups. Specific discovery include quantum annealing (D-Wave), quantum NLP and cybersecurity (Quantinuum), and hybrid quantum-classical models (Rigetti).
Those focused innovations boost the market in niche verticals, eg. D-Wave in transport through optimisation of logistics and scheduling, boost synergies with other technology markets such as genAI (e.g, Quantinuum: Applies quantum NLP for language-based AI systems and cybersecurity, or shape ecosystems through cooperation (eg IonQ partnerships with AWS, Microsoft Azure).
Finally, an unique aspect of the quantum landscape is the proliferation of collaborative ventures. The quantum computing landscape is characterized by extensive collaboration among universities, research institutions, and private companies, fostering significant advancements in the field. These partnerships combine diverse expertise and resources, with a likely consequence of accelerating the development of quantum technologies.
3. Market tipping points
Ultimately, the market will be shaped by a number of tipping points, for which one can already detect some positive, even if still, very noisy, signals at this stage (Table 3).
In terms of economic viability, cost reductions in quantum hardware and platforms are essential for companies to adopt quantum AI. The cost per AI task for quantum is still an order of magnitude higher than for classical computing, assuming the technology enables a robust use case. However, the cost is also falling relatively quickly, suggesting solid performance for quantum AI in the coming years.
In terms of technology breakthroughs, Google’s recent Willow chip show early signs of quantum systems achieving better scaling and error reduction.
Regarding arbitrage opportunities, Quantum AI needs to focus on solving problems where classical AI is slow, expensive or infeasible, such as highly complex optimisation and simulation, while for business transition strategies, the existence of hybrid classical-quantum models offering will allow businesses to experiment with quantum AI without abandoning existing AI investments.
Finally, governments will have a critical role to play in funding and enabling the quantum ecosystem. The regulatory landscapes for Artificial Intelligence (AI), including Quantum AI, differ significantly across China, the European Union (EU), and the United States (US), each reflecting distinct cultural, political, and economic priorities.
A reasonable hypothesis about the likely dynamics is that the combination of substantial government support and agile regulatory adjustments is likely to accelerate quantum AI progress in China. However, strict government control may limit international collaboration and the integration of different perspectives, potentially stifling creative approaches.
While the EU emphasises ethical standards that could lead to responsible quantum AI development, strict compliance requirements could slow innovation and make it difficult for startups and smaller companies to compete.
The US’s flexible, innovation-focused approach may facilitate the rapid development and commercialisation of quantum AI technologies. However, the lack of a consistent regulatory framework could lead to ethical dilemmas, security vulnerabilities and public distrust if not adequately addressed.
The Unknown Unknowns
“Unknown unknowns” refer to uncertainties or challenges that one does not yet realise exist – They will often emerge as quantum and AI technologies evolve. Here are some key potential unknown unknowns in the case of quantum AI:
- Emergent phenomena from quantum and AI synergy: Combining quantum computing with AI could lead to unexpected emergent behaviours or capabilities. We don’t yet fully understand how quantum algorithms could fundamentally reshape AI systems beyond classical limits.
- Quantum data and quantum noise: Classical AI operates on classical data, but we don’t yet know the implications of dealing with “quantum data” generated by quantum systems.
- Quantum black box problems: current AI models (such as deep neural networks) are already black boxes, and adding quantum mechanics could further obscure how decisions or predictions are made.
- New quantum AI security risks: Quantum systems and AI models may introduce unforeseen security vulnerabilities that could be exploited in ways we don’t yet understand. There could be unknown methods of adversarial attacks in quantum-augmented AI systems, where entangled or quantum algorithms make systems uniquely vulnerable.
- Ethical and societal unknowns: biases and quantum effects: Quantum randomness could exacerbate existing ethical problems in AI, or create unexpected new forms of bias.
Table 3 : The quantum AI tipping points
Framing Quantum (AI) Strategy
Let us now summarise the five key findings, from the most certain to the least certain:
- Quantum is real. The promise of quantum computing has been built on seminal milestones such as Feynman’s vision in 1981, Deutsch’s formalisation of quantum computing in 1985, and Shor’s algorithms in the mid-1990s. Since then, companies such as Google and IBM have demonstrated the power of quantum systems, with Google’s “Willow” processor solving problems unattainable by classical supercomputers.
- Quantum markets are just emerging, but may come sooner than expected. The economic viability of quantum computing is not yet proven, but the economics could quickly become attractive in the medium term.
- Transition, more than a complete shift. Hybrid quantum-classical computing is likely to be the transition model to full quantum in the coming years.
- Your AI is your quantum strategy. Advances in quantum capabilities have transformative potential in optimisation, simulation and most AI and machine learning.
- Anticipate responsible/ethical play. The interaction between quantum computing and AI could lead to unexpected breakthroughs, (cyber) risks or even ethical dilemmas.
Many factors traditionally shape a strategy, but the above five elements should serve as a canvas for no regret moves.
Based on the above, corporations should at least build internal quantum literacy and educate employees in AI and quantum and their related synergies, while investing more in hybrid quantum-classical platforms to avoid full reliance on quantum technology at this stage.
Meanwhile, corporations should think about smart experimentation projects, both in AI and in specific vertical projects where quantum can bypass the benefits of classical computing, such as financial optimisation, logistics or drug discovery. Finally, quantum is an entirely new paradigm shift that requires a collaborative approach to ensure the right ecosystem development, as well as regulatory commitment to address the disruptive socio-economic and cybersecurity risks.